7th Presentation | Symbolic regression: re-assessing fluid properties through analytical equations derived exclusively from data with physics-based descriptions | Filippos Sofos
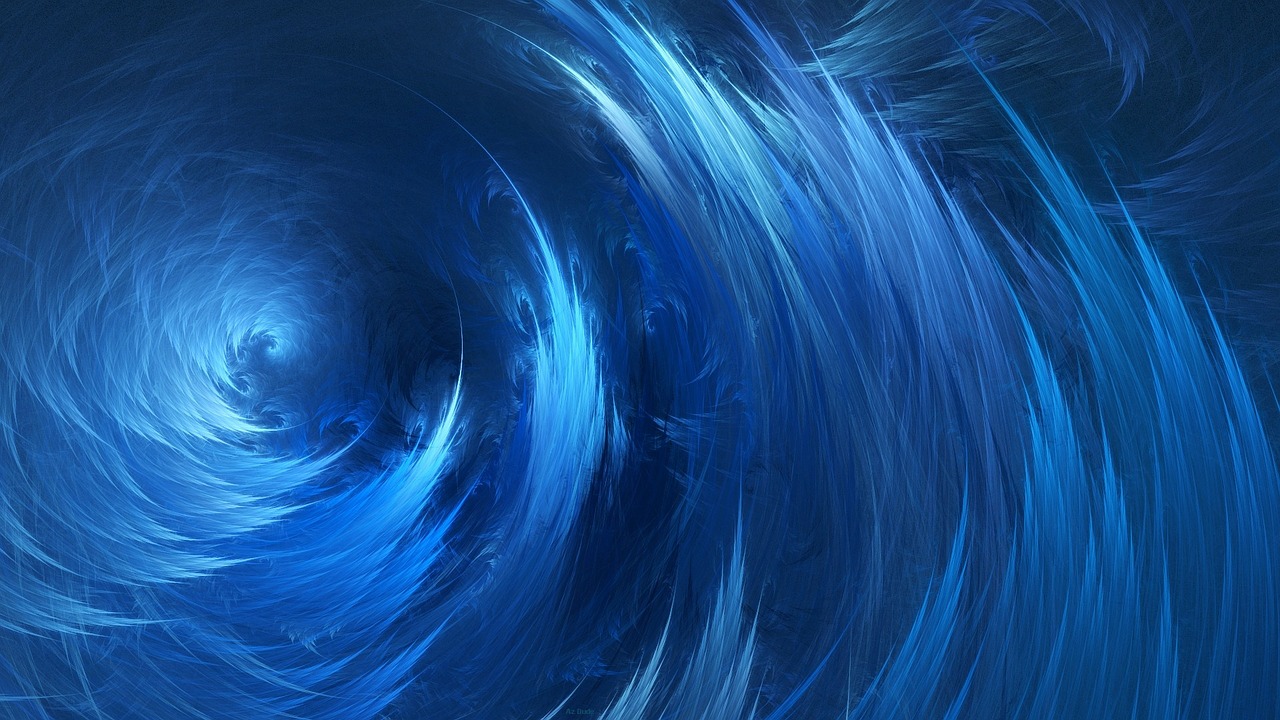
You can watch presentation’s rercording here!
NanoAI welcomes Filippos Sofos who will present his work, titled “Symbolic regression: re-assessing fluid properties through analytical equations derived exclusively from data with physics-based descriptions”.
As always, an open discussion will follow.
When? June 3rd, 15:00 Greece
Where? https://meet.jit.si/nanoai-ncsr
Description: This presentation incorporates Symbolic Regression (SR) to propose simple and accurate expressions that fit to material datasets. SR is a Machine Learning (ML) method, based on formulation derived from Genetic Programming, aiming to unveil hidden physical phenomena by a symbolic expression that fits on a given dataset. The symbolic expression is derived from a potentially infinite search space that can contain any mathematical symbol or function, without any a priori assumption. The incorporation of SR in physical sciences opens the way to develop/replace “black-box” ML techniques with representations that carry the physical meaning and can reveal the underlying mechanism in a purely data-driven approach. Details and applications of SR are to be presented for fluid simulation datasets that refer to the extraction of analytical equations for transport properties (i.e., diffusion coefficient, shear viscosity and thermal conductivity of the Lennard-Jones fluid). Analytical equations of low complexity and error that achieve better or comparable results to well-known microscopic and empirical expressions are shown. The selection of the equations is made on a physics-based analysis, aiming to bind mathematics with hidden aspects of material behavior.
Dr. Filippos Sofos is an Assistant Professor in Computational Condensed Matter Physics, at the Department of Physics, University of Thessaly, GR, since March 2021. He studied Electrical and Computer Engineering at the Democritus University of Thrace (DUTH, 1999), where he also obtained his MSc Diploma in Informatics and Digital Systems (DUTH, 2002). He received his PhD from the Civil Engineering Department, University of Thessaly (U.Th., 2009). The topic of his PhD Thesis was related to the investigation of flows at the nano/micro-scale with the Molecular Dynamics method. He has been involved in many EU and national research projects and his interests include simulations at the nano/meso-scale, multiscale materials simulations, novel materials, solid/fluid interactions, nanofluidics, computational Hydraulics. Current research interests at the ComPhy Lab (comphylab.phys.uth.gr), Department of Physics, U.Th., also include the investigation of machine learning and data science techniques and symbolic regression for materials research with physics-based approaches. He has authored more than 30 journal papers and presented the results in more than 30 international and national conferences.
Subscribe to our mailing list: https://lists.iit.demokritos.gr/mailman/listinfo/nanoai
You can find more info on this upcoming talk as well as on our previous talks at http://nano-ai.iit.demokritos.gr/
Leave a Reply
You must be logged in to post a comment.