18th Presentation | A data lean machine learning approach for the ESTIMATION OF damage extend on composite structures Utilizing Smart Layerwise Spectral Elements | Christoforos Rekatsinas
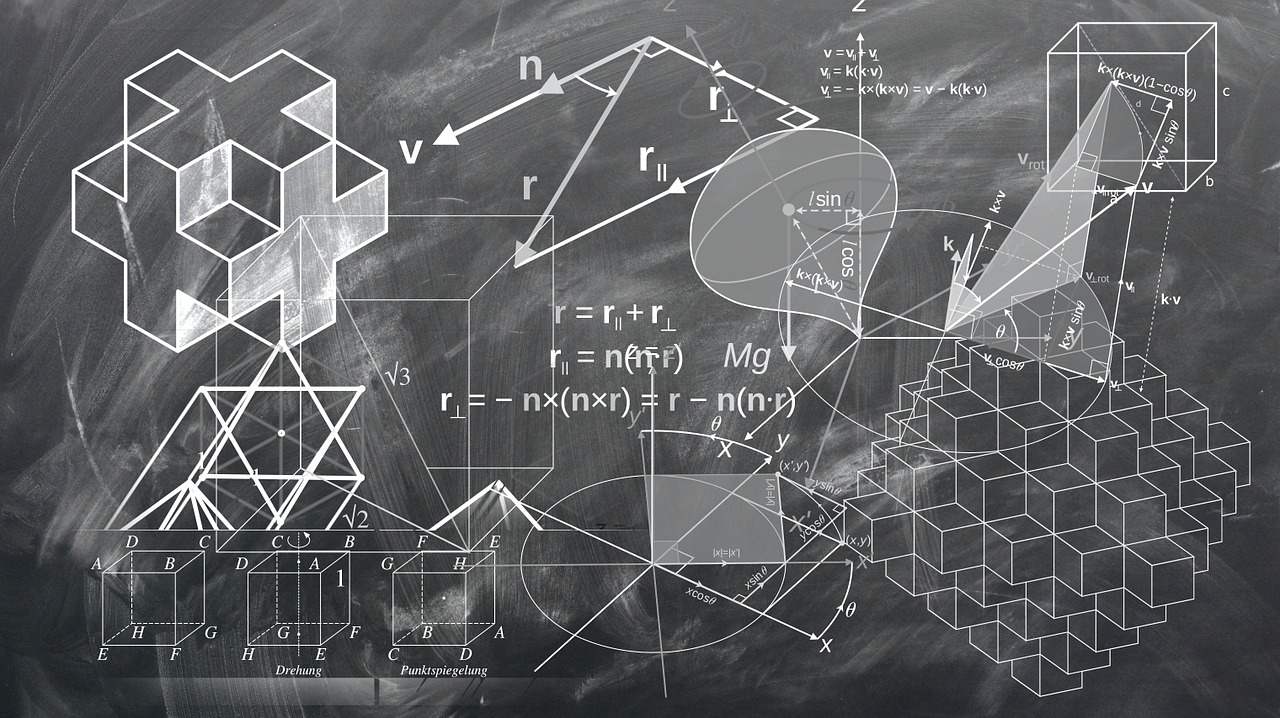
NanoAI welcomes Christoforos Rekatsinas who will present his work, titled “A data lean machine learning approach for the ESTIMATION OF damage extend on composite structures Utilizing Smart Layerwise Spectral Elements”.
As always, an open discussion will follow.
When? December 221st, 15:30 Greece
Description:
The present study discusses the value of a direct interaction between Structural Health Monitoring applications utilizing active piezoelectric sensors and Machine Learning algorithms in order to develop well established Artificial Intelligence driven diagnostics’ tools for composite structures. More specifically, a high-fidelity Time Domain Spectral Finite Element model is developed incorporating physically modeled piezoelectric actuators and sensors as well as mixed order (linear and non-linear) Layerwise Mechanics to simulate efficiently the actual composite structure and all the respective failure modes (fiber, matrix failure and delamination) that could emerge within a composite material. The authors consequently planned a series of damage scenarios which could appear through the thickness of a composite laminate. Afterwards, they simulated the respective virtual experiments of pitch and catch technique corresponding to the aforementioned scenarios by applying an actuating Guassian pulse and acquiring the respective responses of three sensors. Part of these data were then used as input to train different machine learning models predicting the composite material damage and classifying the damage extend(output). We examine different approaches such as (KNN and LSTM) for representing the data to feed these machine learning models and present the very promising first findings regarding the effectiveness of the prediction, based on a cross-validation process on the generated simulation data.
Christoforos Rekatsinas has been an associated researcher at the Institute of Informatics & Telecommunications of NCSR Demokritos since January 2023. He holds both a Diploma of Mechanical & Aeronautics Engineering (2010) and a Ph.D. (2016) from University of Patras in the field of computational mechanics of composite and smart materials. He has worked as a post-Doctoral researcher in the Department of Mechanical & Aeronautical Engineering of University of Patras attaining a technical and managerial experience of seven years in European (CleanSky, FP7) and Domestic (Research – Create – Innovate) research projects. Awarded an Hrakleitous II doctoral fellow in 2010, a best PhD thesis for its contribution on the Aerospace Industry from the CleanSky Academy in 2018. Now his work focuses in the field of Reinforcement Learning for Structural Design applications with focus on composite and smart materials and on the utilization of Machine Learning envisioning to facilitate the Structural Damage Indicators of Composite Structures. He joined the INSANE group led by Dr. Giannakopoulos, where he now explores the interoperability of ML and Natural Sciences. Finally, he is currently engaged in one European Project, with interests spanning from AI tools combating misinformation and terrorism in media (AI4TRUST, with project numbers 101070190), one to the field of AI technology transfer (AI4Copernicus, project number 101016798) and in one where he acts as PI funded by Science Agora for the development of an Autonomous Surface Vehicle for the clean up of seas and lakes.
Subscribe to our mailing list: https://lists.iit.demokritos.gr/mailman/listinfo/nanoai
You can find more info on this upcoming talk as well as on our previous talks at http://nano-ai.iit.demokritos.gr/