4th Presentation | Development of coarse-grained molecular simulation models invoking machine learning techniques | Dimitris Nasikas
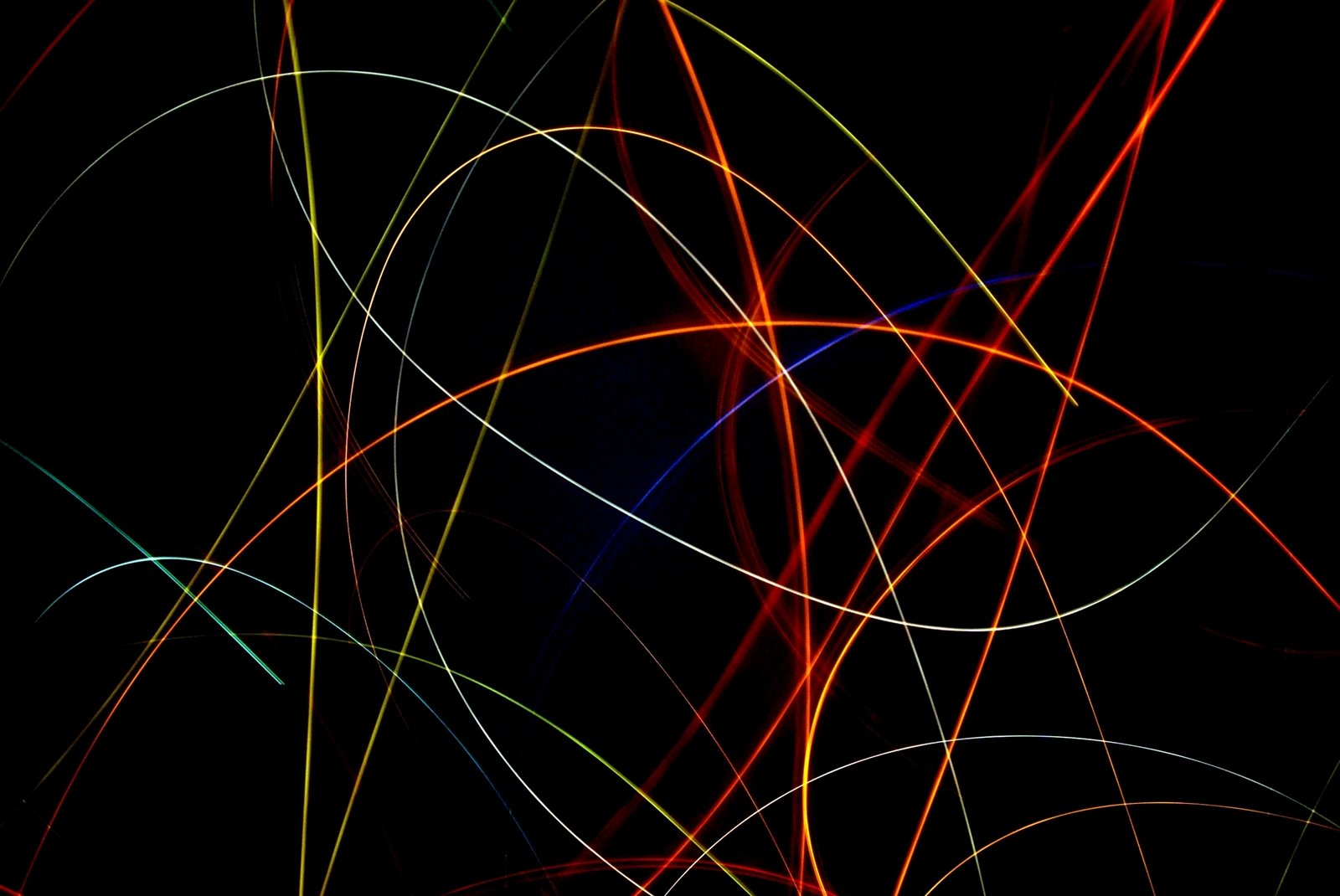
NanoAI welcomes Dimitris Nasikas, who, in the context of his M.Eng thesis, will present his interdisciplinary work on neural networks applied for molecular simulations, titled “Development of coarse-grained molecular simulation models invoking machine learning techniques”.
As always, an open discussion will follow.
When? October 1st, 16:00 Central European Time. / 15:00 Greece
Where? https://meet.jit.si/nanoai-ncsr
Description: Molecular simulation is a particularly efficient and reliable means for the study of molecular mechanisms that underlie the macroscopic behavior of materials and for the prediction of their properties as well as for the design of new materials. The broad spectra of length- and time-scales present in complex chemical systems such as the macromolecular ones, necessitate the implementation of hierarchical multi-scale approaches. Within this scope, the development of appropriate coarse-graining schemes are often nessessary. These schemes involve the substitution of groups of atoms by single interaction sites, thereby reducing the number of the system’s degrees of freedom, while at the same time maintaining the ones that are important for the description of the mechanisms or processes under study. Coarse-graining approaches involve the mapping from the atomistic to a coarse-grained level and equilibration at the coarse-grained level and reverse mapping back to the atomistic representation. The present work focuses on the development of coarse-graining strategies using machine learning techniques. Variational Autoencoders (VAEs) are used to determine the mapping from the atomistic level to a coarse-grained one and also to reconstruct the initial configurations. The VAE uses as training data snapshots of molecular dynamics trajectories of selected chemical systems. The training data consist of the coordinates and the forces of the atoms at each snapshot. The effect of various training processes as well as the choices of the VAE ‘s hyperparameters on the extracted results are thoroughly investigated for selected test chemical systems.
Dimitris Nasikas is currently a last year Chemical Engineering student at the National Technical University of Athens completing his thesis in collaboration with NCSR Demokritos. His thesis is focused on the development of coarse-grained models for molecular simulations using machine learning methodologies.
Subscribe to our mailing list: https://lists.iit.demokritos.gr/mailman/listinfo/nanoai
You can find more info on this upcoming talk as well as on our previous talks at http://nano-ai.iit.demokritos.gr/
Leave a Reply
You must be logged in to post a comment.