2nd Presentation: Hybridizing AI and domain knowledge in nanotechnology | Antonis Stellas
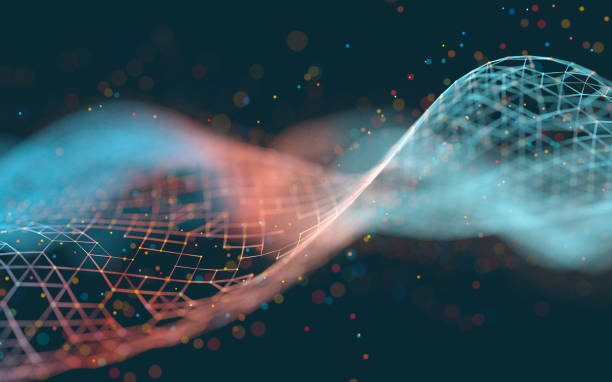
Review the Presentation Video & Slides and related publication
Manufacturers and researchers who are working with the quantitative characterization of nanostructure morphologies often deal with the challenge of understanding the link between the structure of their surface and the respective property that they want to achieve. This link is difficult to understand and model due to high theoretical complexity and time cost of simulation. In this presentation, we want to show you our attempt of investigating an alternative (data-driven) solution in the example of surface roughness effects for predicting wetting behavior. We use ML methods to connect the structural and functional parameters to nanostructured surfaces with roughness.
Even though the ML models are characterized as black boxes, our machine learning workflow combined with the domain knowledge could help to make the box a bit more transparent and actually help us gain insights into our problem. In this presentation, we will explore the world of rough surfaces and their effect on wetting behavior. How are ML models useful? How are we making our workflow more transparent and useful?
Antonis Stellas holds a diploma in applied mathematics and physics as well as a master’s degree in nanotechnology. In the last two years, he has been living in Eindhoven where he had the opportunity of doing data science projects with companies’ around Netherlands. He recently acquired his Professional doctorate in Data science. He is passionate on working and learning more about data science and nanotechnology. In his free time, he plays tennis and since corona, he started learning the guitar.
Leave a Reply
You must be logged in to post a comment.