16th Presentation | Modelling Gas Sorption in Glassy Polymeric Membranes: A Hybrid Machine Learning & Equation of State (EoS) Approach | Hasan Ismaeel
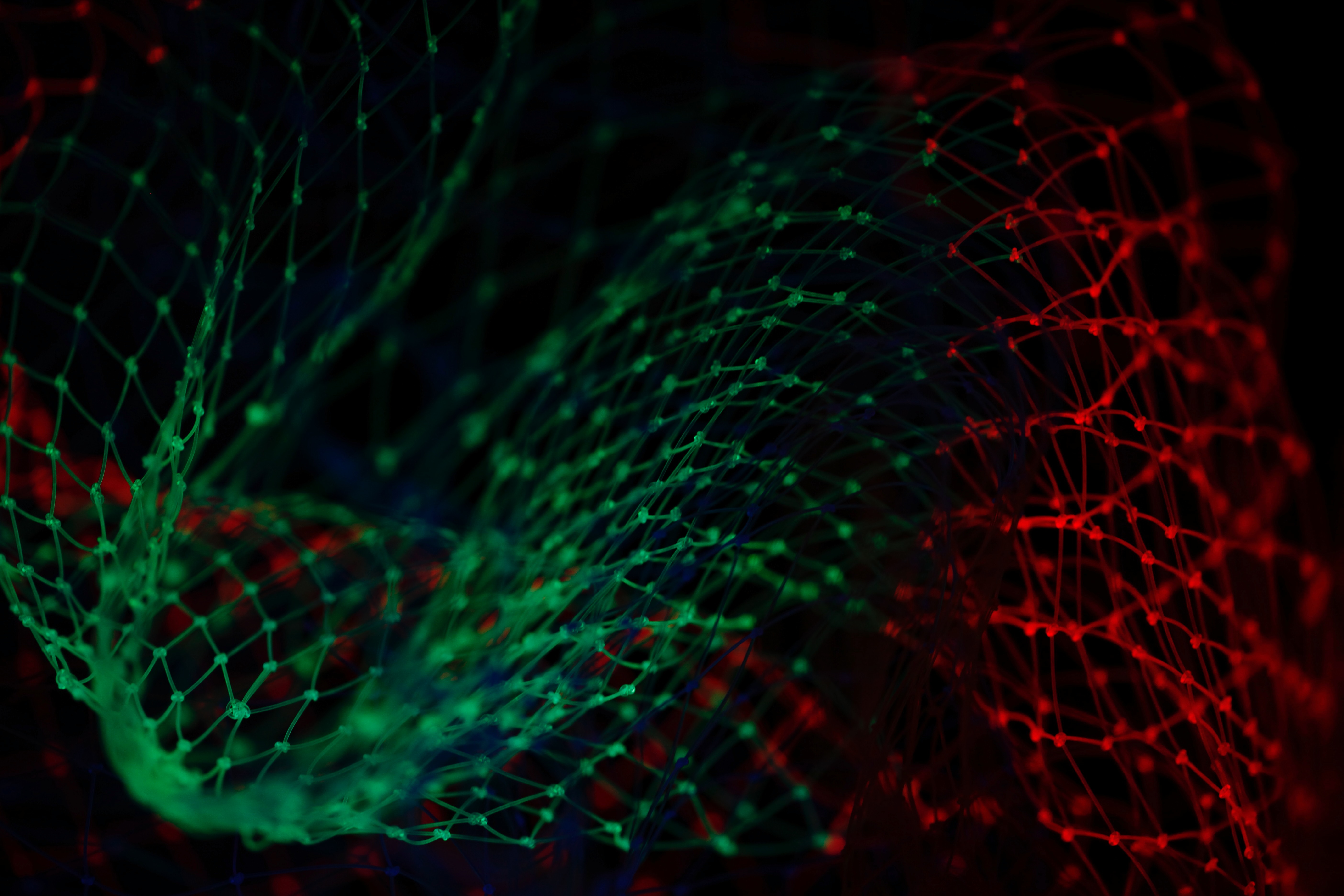
You can watch presentation’s recording here!
NanoAI welcomes Hasan Ismaeel who will present his work, titled “Modelling Gas Sorption in Glassy Polymeric Membranes: A Hybrid Machine Learning & Equation of State (EoS) Approach”.
As always, an open discussion will follow.
When? October 5th, 15:00 Greece
Description:
Polymeric membrane-based gas separation processes have been garnering increasing attention throughout the years as a promising alternative to the incumbent energy intensive, thermal separation processes used today. A systematic understanding of what drives the thermodynamics of such systems, particularly in solubility-based separation processes, is a key component in the development of high performing polymeric membranes.
Since the introduction of the Non-Equilibrium Thermodynamics for Glassy Polymers (NET-GP) framework, equations of state (EoS) have become a staple tool in the modelling of the gas solubility (i.e., the thermodynamic factor) in glassy polymeric membranes. EoS derived from statistical mechanics, such as the Sanchez and Lacombe Lattice Fluid Theory (LF) and the Perturbed-Chain Statistical Associating Fluid Theory (PC-SAFT) have been built to explicitly account for the non-idealities introduced by the polymer molecules and was used successfully in correlating the gas sorption behaviour of many systems. However, the applicability of EoS is highly restricted by the availability of the pure and mixture parameters, thereby limiting its predictive capabilities for some systems.
By utilizing machine learning regression techniques, a functional relationship can be developed between the EoS parameters and the chemical structure of the components. In this work, we have employed machine learning, alongside group contribution (GC) methods, to determine the pure polymer parameters of EoS. In addition, we have investigated several techniques of parameterizing high glass transition temperature (Tg) polymers for the PC-SAFT EoS, which has not been explored thoroughly in literature. Moreover, we have also probed some of the predictive capabilities of the PC-SAFT EoS, especially in areas where the LF model fails, in particular, systems exhibiting hydrogen bonding, and predicting the gas sorption behaviour in co-polymers across the monomer composition range.
Hasan is a 2nd year PhD Chemical Engineering student at the University of Edinburgh, supervised by Prof. Maria Grazia DeAngelis. Hasan has obtained his bachelor’s degree from the University of Leeds (2015) in Chemical Engineering, and his master’s degree from Imperial College London in Advanced Chemical Engineering (2019). In his PhD thesis, Hasan is investigating different means of modelling the gas solubility in glassy polymeric membranes, through the aid of machine learning, physics-based models like equations of state, or a hybrid of both.
Subscribe to our mailing list: https://lists.iit.demokritos.gr/mailman/listinfo/nanoai
You can find more info on this upcoming talk as well as on our previous talks at http://nano-ai.iit.demokritos.gr/